How To Leverage AI & Machine Learning To Maximize Manufacturing Efficiency
1. Artificial intelligence and machine learning are not the same
Artificial intelligence (AI) and machine learning (ML) have become prominent buzzwords, attracting more attention than ever before. While these terms are often used interchangeably, it's important to recognize their distinctions to fully grasp their potential value.
AI serves as a broad category encompassing various technologies designed to tackle complex problems and emulate human cognitive abilities. It encompasses tasks typically associated with human intelligence, such as reasoning, problem-solving, and learning from experiences.
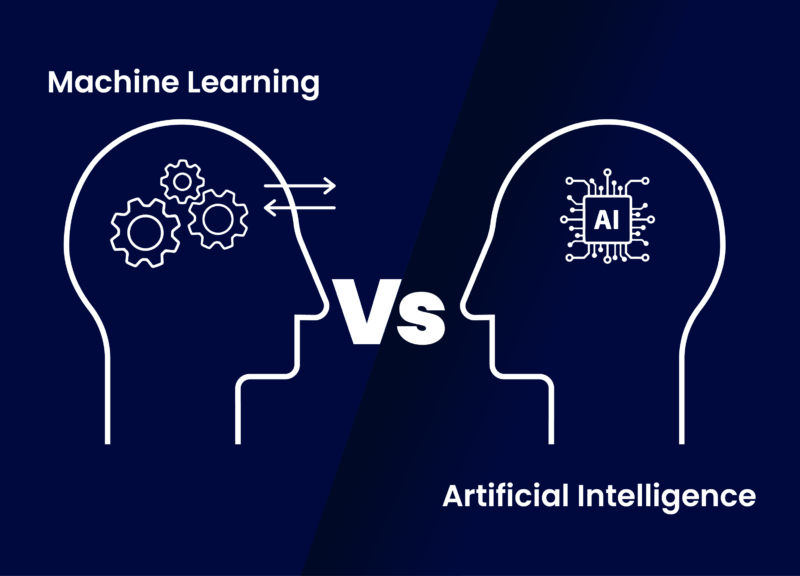
On the other hand, machine learning operates as a subset within AI. ML algorithms autonomously ingest and analyze new data, identifying patterns and using this knowledge to make informed decisions without requiring explicit programming. By continually learning from data, ML systems minimize the need for human intervention.
In the realm of business, where data flows from multiple sources, ML plays a crucial role. By processing and analyzing data, ML algorithms uncover patterns, anomalies, and trends, empowering executives and managers to make informed decisions and optimize operations effectively.
2. Challenges and Drawbacks of Traditional Manufacturing Methods
Traditional manufacturing processes, while effective in their time, come with several disadvantages compared to modern, AI-driven manufacturing methods:
- Lack of Efficiency: Traditional manufacturing processes often rely on manual labor and outdated machinery, leading to lower productivity and higher production costs compared to AI-driven automation.
- Limited Flexibility: Traditional manufacturing setups are typically less flexible and adaptable to changes in production requirements or market demands. Adjusting production schedules or reconfiguring production lines can be time-consuming and costly.
- Higher Error Rates: Manual processes in traditional manufacturing are prone to human errors, resulting in lower product quality, higher scrap rates, and increased rework costs. AI-driven quality control systems can significantly reduce these errors and improve product consistency.
- Reactive Maintenance: Traditional manufacturing facilities often practice reactive maintenance, where equipment is repaired only after it breaks down. This approach leads to unplanned downtime, higher maintenance costs, and decreased overall equipment effectiveness (OEE).
- Limited Data Insights: Traditional manufacturing processes typically generate limited data and lack real-time insights into production performance and quality metrics. Without access to comprehensive data analytics, manufacturers may struggle to identify areas for improvement and make informed decisions.
- Higher Operational Costs: Manual labor, inefficient processes, and reactive maintenance practices in traditional manufacturing contribute to higher operational costs compared to AI-driven manufacturing methods. These higher costs can erode profit margins and hinder competitiveness.
- Slower Time-to-Market: Traditional manufacturing processes often have longer lead times from product design to production compared to modern, agile manufacturing approaches enabled by AI and machine learning. This slower time-to-market can delay product launches and hinder competitiveness in fast-paced markets.
- Environmental Impact: Traditional manufacturing methods may have a higher environmental impact due to higher energy consumption, increased waste generation, and higher emissions. AI-driven manufacturing methods can help optimize energy usage, reduce waste, and minimize environmental footprint.
Overall, the cons of traditional manufacturing include lower efficiency, limited flexibility, higher error rates, reactive maintenance practices, limited data insights, higher operational costs, slower time-to-market, and a potentially higher environmental impact compared to modern, AI-driven manufacturing methods.
3. Maximizing Manufacturing Efficiency: Leveraging AI & Machine Learning with DocuWare
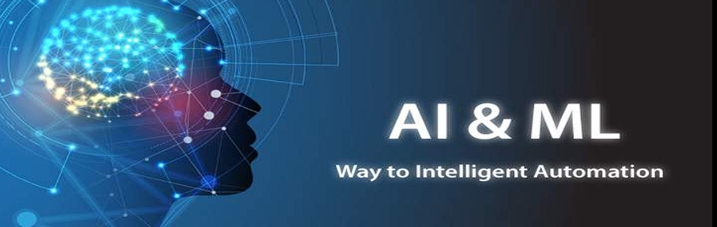
In today's rapidly evolving manufacturing landscape, staying competitive requires embracing advanced technologies that optimize operations and drive innovation. Among these technologies, artificial intelligence (AI) and machine learning (ML) stand out as game-changers, offering manufacturers unprecedented capabilities to enhance efficiency, productivity, and quality. One such platform leading the charge in this transformation is DocuWare, providing comprehensive solutions tailored to the needs of modern manufacturing facilities.
AI and ML have the potential to revolutionize every aspect of manufacturing, from predictive maintenance and quality control to supply chain management and product design. By harnessing the power of AI algorithms and ML models, manufacturers can unlock valuable insights from vast amounts of data, streamline processes, and make data-driven decisions that drive business success.
Predictive maintenance is one area where AI and ML shine in manufacturing. By analyzing equipment data in real-time, AI-powered predictive maintenance systems can anticipate potential failures before they occur, enabling proactive maintenance actions that minimize downtime and extend equipment lifespan. This proactive approach not only reduces maintenance costs but also enhances overall equipment effectiveness (OEE) and improves production efficiency.
Quality control is another critical aspect of manufacturing that benefits greatly from AI and ML technologies. Traditional manual inspection processes are time-consuming and prone to errors, leading to inconsistencies in product quality. AI-driven vision systems, integrated with DocuWare, can accurately detect defects and anomalies in real-time, ensuring that only high-quality products reach the market. By automating quality control processes, manufacturers can reduce scrap rates, improve yield, and enhance customer satisfaction.
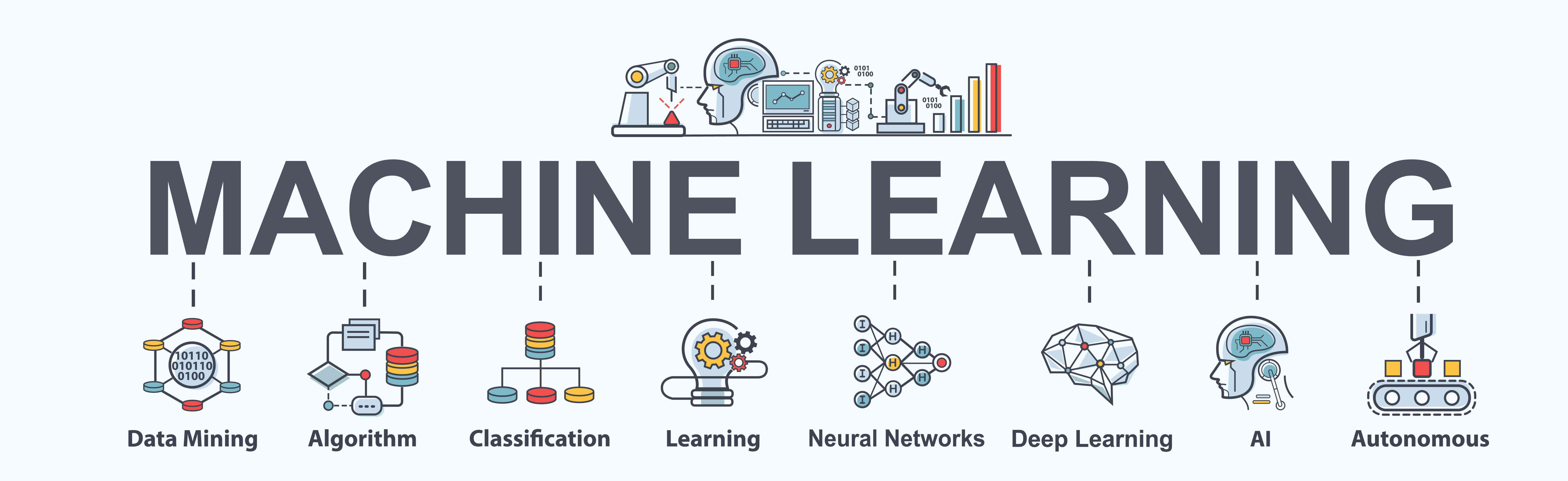
In addition to predictive maintenance and quality control, AI and ML enable manufacturers to optimize production processes, enhance supply chain management, and accelerate product development. AI algorithms can analyze production data to identify inefficiencies, optimize resource allocation, and streamline workflows. By leveraging AI-driven demand forecasting and supply chain optimization, manufacturers can minimize inventory costs, reduce stockouts, and improve overall supply chain efficiency.
DocuWare plays a pivotal role in enabling manufacturers to leverage AI and ML effectively. As a leading provider of document management and workflow automation solutions, DocuWare offers robust AI-powered capabilities that streamline document-intensive processes, enhance collaboration, and improve data visibility. With DocuWare's intuitive interface and seamless integration with AI and ML technologies, manufacturers can harness the full potential of these advanced tools to drive continuous improvement and innovation.
In conclusion, the integration of AI and ML technologies in manufacturing, coupled with DocuWare's comprehensive solutions, presents a transformative opportunity for manufacturers to optimize operations, increase productivity, and achieve sustainable growth. By leveraging AI and ML with DocuWare, manufacturers can stay ahead of the competition, adapt to changing market dynamics, and thrive in the digital age of manufacturing.
News & Events
Keep up to date
- 06Aug
Ricoh Achieves 'Gold Provider' Partner Status with CISCO in the Asia-Pacific Region
- 28Jun
Ricoh named in TIME World's Most Sustainable Companies of 2024
- 19Jun
Register for the 5th Color Free Printing with Ricoh Pro C7500
- 19Jun
Ricoh named in Asia-Pacific Climate Leaders list for the third consecutive year